What Are AI Agents? New Era of AI Autonomy
Artificial intelligence is evolving at an unprecedented pace, and we have entered the phase that many call the "third wave" of AI evolution. While we are already used to traditional AI and generative AI tools, which have revolutionized how we analyze data, access information, generate creative outputs, and even create art, now we're witnessing the emergence of something much more complex - Agentic AI. Agentic AI is the new level of AI sophistication where systems, known as AI agents, are designed to be able not only to analyze data, respond to prompts, and generate content but also to autonomously think, decide, and act.
The concept of autonomous AI agents is not new. However, the general consensus in the past was that most AI-based systems simply did not follow instructions with sufficient quality to perform critical business functions or decisions without human supervision. But as AI continues to improve, we are approaching a point where the results produced by AI reach such a level of quality that humans no longer need to directly manage the functions of AI-driven software. Instead, they can simply let them act on their own. And while fully Agentic AI, the one that is highly autonomous, adaptive, and capable of self-learning without predefined boundaries, is still an emerging field in its infancy, we are already seeing how simpler, task-focused AI agents are widely implemented today.
So What Exactly Are AI Agents?
Basically, AI agents are systems that can perceive their environment, make decisions, and take actions to achieve specific goals—either autonomously or semi-autonomously. They can come in different types that vary in complexity and capabilities, but they all share some common characteristics. They all generally collect and process input from their environment through sensors or input mechanisms, use moderate to advanced reasoning mechanisms to evaluate the best course of action and perform tasks. Many AI agents can also adapt to changes in their environment in real time, improving their performance through feedback and learning.
The ability of AI agents to act autonomously is what distinguishes them from traditional and generative AI systems. The latter have limitations when it comes to performing complex tasks or chaining multiple steps together. Agentic AI takes things a step further. It can analyze situations, make decisions, and perform tasks without requiring constant human oversight or predefined step-by-step guidance.
Here are the main features that distinguish AI agents from traditional AI or generative AI models:
1. Autonomy: They act independently without human intervention. They adapt to changing conditions and can make decisions based on goals and data. They require less supervision and are more dynamic.
2. Adaptability: Advanced AI agents can learn from experience and continuously adjust their behavior, unlike traditional systems that need to be retrained to deal with new situations or require human intervention.
3. Goal-orientation: They focus on achieving evolving long-term goals, planning actions, and adjusting strategies. In contrast, traditional AI systems are often task-specific and lack long-term adaptability.
How do AI Agents work?
Imagine you prompt an AI agent to "book the best-rated hotel near the city center of Destination X for Z dates under $200 per night". The AI agent searches various hotel booking platforms, filtering results based on proximity to the city center, user reviews, and price range. It evaluates the options and selects the hotel that offers the highest combination of rating, location, and price within the specified budget. The agent connects to the hotel's booking API, fills in the required details (e.g., check-in/check-out dates, number of guests), and reserves the room. If the agent finds no hotels that meet all criteria (e.g., the price exceeds $200), it notifies the user and suggests alternatives, such as increasing the budget or expanding the search area. Once the user confirms or adjusts preferences, the agent finalizes the booking and sends confirmation details to the user.
This example reflects how most autonomous agents follow a specific workflow when performing assigned tasks. They start by receiving instructions or goals through a user-specified prompt. Then they break it down into smaller, manageable steps and move seamlessly from one task to the next, much like a human user, without requesting additional human input in between steps. If necessary, they collect data, such as conversation logs or information from the Internet. In some cases, they collaborate with other AI systems to share data or ideas. Using their knowledge and the data they have collected, they complete tasks with the help of the tools available to them. Throughout the process, they check their progress by seeking external feedback and inspecting their own logs. If the goal is not fully achieved, they adapt by creating and completing additional tasks until they achieve the desired outcome. The basic idea behind agentic interaction is that the system understands your goal and works towards it on its own. However, humans still play a role in monitoring the process and intervening when necessary.
What are the main types of AI agents?
Based on complexity and capabilities, here are the main types of AI agents and how they are applied in real-world scenarios. It is worth mentioning that, in practice, advanced AI systems often combine features from multiple types of agents to achieve their goals.
Simple Reflex Agents: These basic long known AI systems operate on a condition-action rule. They don't learn or adapt; they simply react based on the information they have at the moment, so they are suitable for simple tasks that don't require extensive training. A chatbot that resets passwords when it detects certain keywords in a conversation or a thermostat that adjusts the heat based on the room's current temperature are examples of simple reflex agents.
Model-Based Reflex Agents: These are also basic AI systems similar to simple reflex agents but they maintain an internal representation of their world to evaluate actions and predict outcomes. For instance, a robotic vacuum that maps your home keeps track of the areas it has already cleaned and identifies spots that still need cleaning. This internal model helps it decide where to go next.
Goal-based agents (also called rule-based agents): These are advanced AI systems that consider how their actions will help achieve designated goals rather than simply reacting to stimuli. Such agents aim to choose the most efficient path. They are suitable for performing complex tasks. For instance, Smart Home AI systems that need to ensure energy efficiency can evaluate room usage and adjust lights, HVAC systems, and other devices to meet their energy-saving objectives.
Utility-Based Agents: These complex AI systems go beyond achieving simple goals. They weigh multiple options and optimize for the best possible outcome based on measurable benefits or satisfaction levels in each scenario. The agent then selects the action that maximizes the utility or provides the best outcome for the user. For example, customers can use a utility-based agent to find the optimal balance between cost and travel time. Or, for instance, a self-driving car that evaluates different routes based on travel time, road conditions, safety, and fuel efficiency.
Learning Agents: These complex AI systems continuously learn from previous experiences to improve their results. Using sensory input and feedback mechanisms, the agent adapts its learning element over time to meet specific standards. On top of that, it uses a problem generator to design new tasks to train itself from collected data and past results. These can be, for instance, a recommendation system that improves its suggestions or a spam email filter that continuously improves its accuracy based on user feedback.
Are there real world use cases of AI agents?
AI agents are already taking on complex tasks and improving efficiency. Virtual assistants like Alexa, Siri, and Google Assistant are examples of basic AI agents. These AI agents process users' commands, learn from their behavior, and autonomously perform tasks like setting reminders, checking the weather, or playing music, all with minimal user effort. Beyond simple tasks they can manage the user's entire digital ecosystem, integrating with other apps, such as apps controlling smart home devices, etc. Still, these AI agents aren't yet fully autonomous or capable of complex decision-making and action-taking on their own.
Autonomous vehicles are another example of AI Agents already in use. These AI agents can navigate traffic, communicate with traffic management systems, make driving decisions, and adapt to or adjust routes based on real-life road conditions or obstacles. For instance, Waymo self-driven cars are designed for zero passenger interaction during the ride. Passengers sit in the back and cannot intervene. They already operate in cities like Phoenix, San Francisco, and Los Angeles. And they perfectly display "agentic" AI in action, i.e. where the vehicle makes decisions and takes actions autonomously, without any human input. But even though agents like Waymo can drive themselves, they still operate under specific conditions (like particular cities or routes), and human oversight is needed for certain situations.
AI agents are also transforming industries such as healthcare, logistics, e-commerce, finance, and more. These industry-specific AI solutions are known as Vertical AI agents. In healthcare, AI agents help diagnose diseases, analyze medical images, and manage patient care. For example, an agent like Viz.ai can detect a stroke through a brain scan and notify doctors in real time, allowing for faster treatment decisions. In finance, AI agents analyze market trends, manage investments, detect fraudulent activity, and provide personalized financial advice. For example, Kavout is an AI agent that autonomously analyzes market data and makes real-time decisions about stock trading. It evaluates financial indicators and market conditions to execute trades that meet the user's predefined goals without requiring human intervention in the process.
While most AI agents we see today can still be considered more basic and not fully autonomous AI agents, they still represent a major shift of moving beyond traditional assistant roles into more autonomous decision-making and task execution. Their autonomy works best in collaboration with human professionals, who can make final decisions based on AI recommendations. But they are paving the way for the final goal of fully Agentic AI.
Bottom Line
AI agents appeared as part of the broader evolution of AI, which marks a significant shift in how we interact with technology—moving from simple automation or generation to complex, autonomous decision-making systems that can truly act as partners in our daily lives. The future of AI isn't just about responding—it's about taking intelligent, independent actions. With such autonomy, adaptability, and real-time decision-making capabilities, these advanced AI systems are set to become indispensable in homes, businesses, or across a range of industries like logistics, e-commerce, or healthcare. The question now is not whether AI agents will transform industries but how quickly their impact will spread.
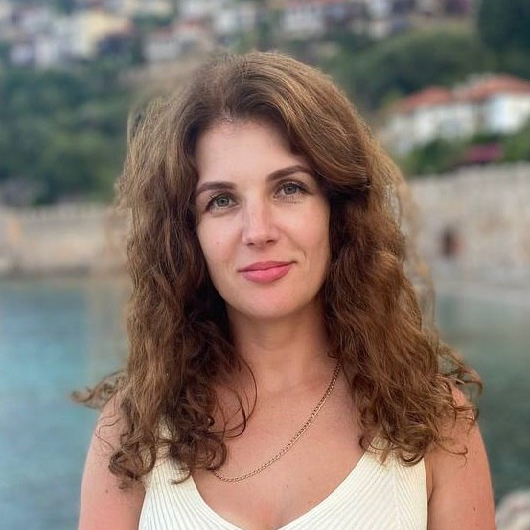